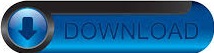
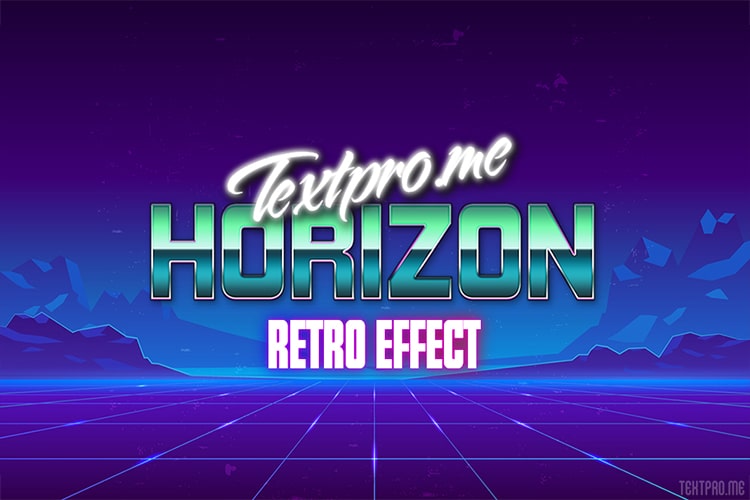
Keeping in mind the aforementioned issues, this thesis first enlists a systematic overview This prompts the deployment of DL for HSI Classification (HSIC) which revealed good performance. In the last few years, Deep Learning (DL) has been substantiated as a powerful feature extractor that effectively addresses the nonlinear problems that appeared in a number of computer vision tasks. Notably, the complex characteristics i.e., the nonlinear relation among the captured spectral information and the corresponding object of HSI data make accurate classification challenging for traditional methods. Hyperspectral Imaging (HSI) has been extensively utilized in many real-life applicationsīecause it benefits from the detailed spectral information contained in each pixel. Using a combination of MV and IP, followed by the use of special classification algorithms, helps to have more efficiency in the detection of defects in harvested products.
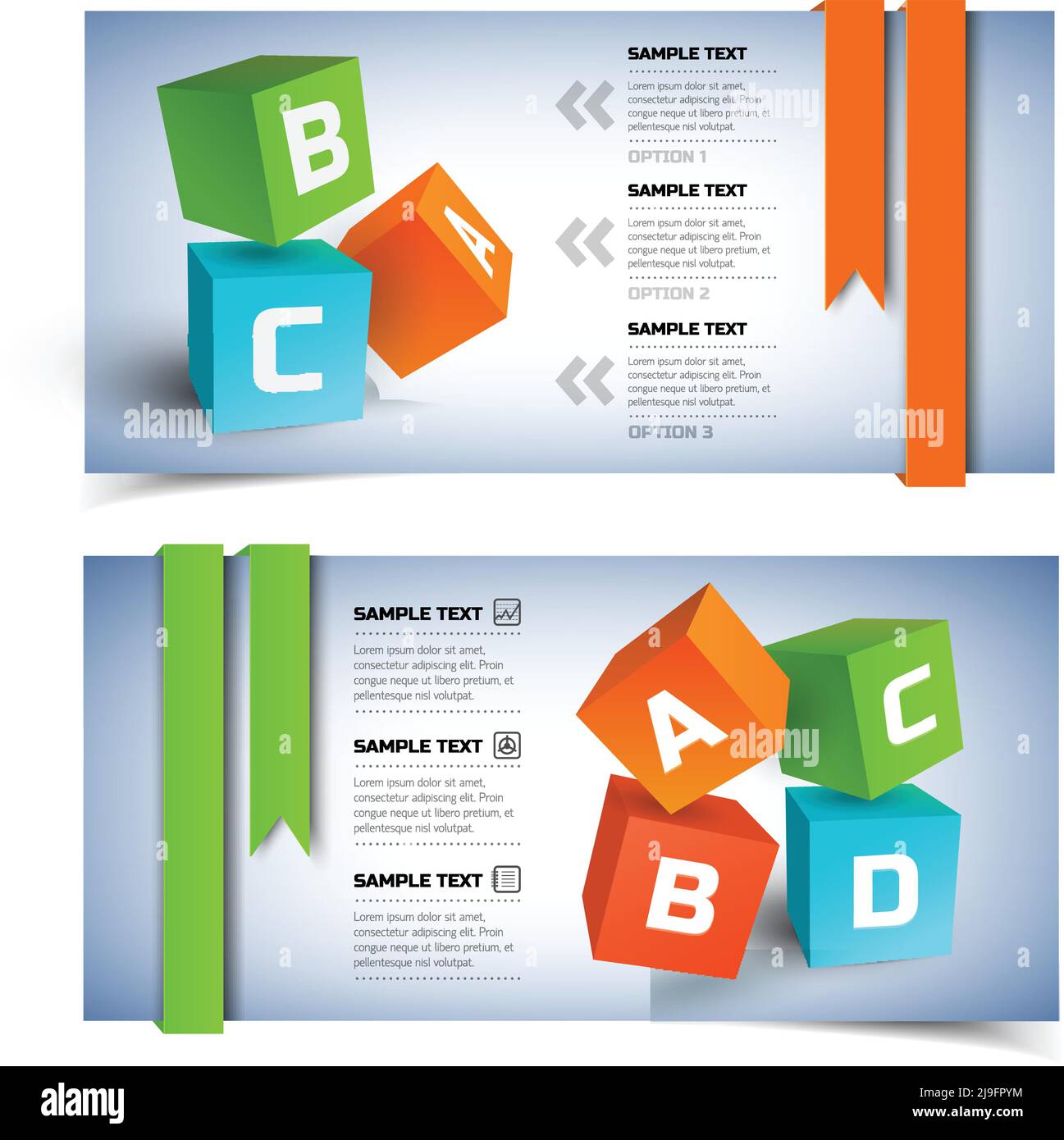
Many classification algorithms allow us to categorize products based on the quality and type of their defects.
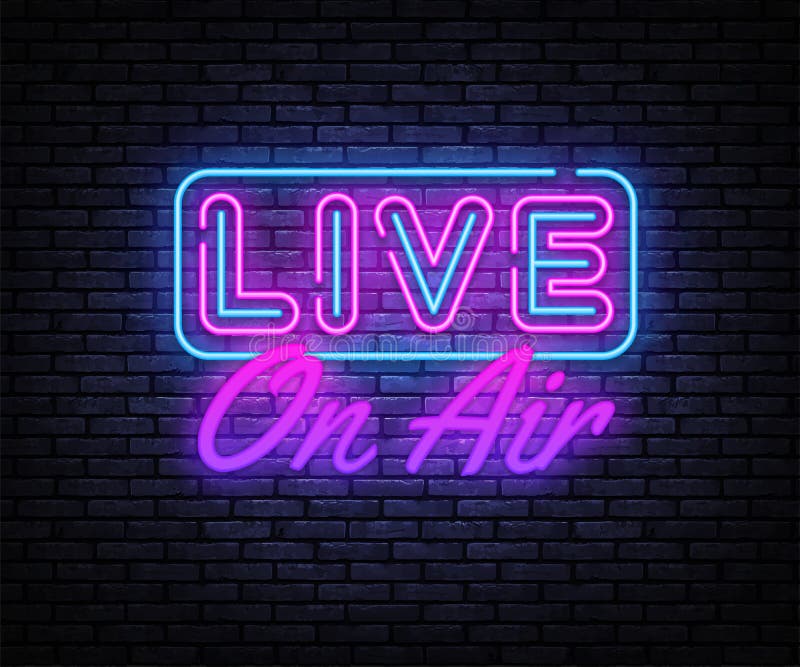
In the next step, they can be classified based on the detected defection. In the first step, as long as agricultural products are harvested, in a suitable condition with good illumination, they are photographed by special cameras and evaluated by the IP science. In this review, the importance of quality inspection in the agricultural industry and its effect on worldwide markets are highlighted and the ways which help to categorize the products by their defections. Defects generally appear due to insect damage, scarring, product decay, and so on. Today in the agricultural industry, many defects affect production efficiency this paper aims to show how the combination of machine vision (MV) and image processing (IP) helps to detect the defective areas of products.
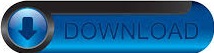